There is considerable excitement about the potential of AI to deliver more accessible, efficient, and high-quality healthcare; alongside concern about data privacy, bias, and how these new tools will be used in clinical practice.
The key to realising the benefits and addressing the concerns is the adoption of standards for the development and implementation of AI by manufacturers and their customers. And the good news is that standards are both available and developing rapidly, says Dean Mawson, clinical director and founder of DPM Digital Health Consultancy.
There’s considerable interest in the potential uses of AI in healthcare at the moment; but there is also concern about the possible risks that it could pose.
Challenges include questions about data privacy and algorithmic bias, how we can make sure that AI tools are subject to robust validation and testing processes, and how to make sure they are used safely in a clinical setting.
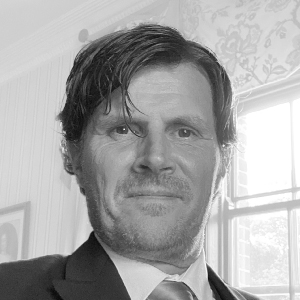
To address these issues, manufacturers will need to be transparent about their data models and the way their algorithms are trained and validated. There will also need to be more education and training for the people who procure and use these tools.
Building trust
However, that will only take us so far. Manufacturers are, understandably, keen to protect their intellectual property – and some AI operates as a ‘black box’ around which we can only see inputs and outputs.
At the same time, busy healthcare organisations, clinicians and patients need to understand the fundamentals, but are never going to be experts in such a complex area. So, how do we secure the adoption of AI in this environment, and make sure its risks are properly managed?
The key is going to be ‘trust’ which the Oxford English Dictionary defines as: ‘a firm belief in the reliability, truth, or ability of someone or something’. And one way in which other sectors, from airlines to engineering and med tech, build trust is through regulation.
Few people in the world really understand how a plane is built or a nuclear power plant operates. Instead, we trust they are safe because they are highly regulated, and operate to well understood, international standards.
Standards for AI in healthcare
Since the Covid-19 pandemic, which saw a rapid acceleration in the take-up of health tech of all kinds, there has been growing interest in standards for AI in healthcare.
In the UK, the starting point is DCB0160 and DCB0129, which date back 15 years to a programme to encourage health tech vendors and their customers to take a ‘safety approach’ to the design, development, deployment and use of digital health systems.
DCB0160 requires trusts to risk assess any customisations and reconfigurations, to determine whether they are good to go live and DCB0129 requires vendors to carry out a risk assessment on their product.
Both should be very familiar, as compliance with these standards is mandatory under the Health and Social Care Act 2012. Then, we have BS ISO/IEC 30440 and BS ISO/IEC 42001.
These are international standards developed by experts from 50 countries, led by the British Standards Institution, and they provide a validation framework and a management system for AI in healthcare.
BS ISO/IEC 30440 is designed to help manufacturers to risk assess medical technology using machine learning and to mitigate any hazards found. While BS ISO/IEC 42001 is designed to help organisations to create a management system to implement and govern this technology effectively.
User friendly – up to a point!
The BSI and its experts have worked hard to make these standards user-friendly. For international standards, they are written in lay-person’s terms and come with examples for some of the clauses, indicating how to apply them.
Even so, it’s been recognised that adopting these standards is not straightforward, and the University of York has been commissioned to develop a safety assurance framework to help manufacturers and deploying organisations.
This is underpinned by an established process known as the assurance of machine learning for use in autonomous systems, or AMLAS. Effectively, the University is working out how to apply this to healthcare.
Challenges to using standards in practice
So, we have some standards for the development and deployment of health IT systems generally and AI tools specifically, and the start of a structure for applying them, but there’s no doubt that we are at the start of a journey.
As we learn more about AI in healthcare, we’re going to need to revise the standards and review our governance arrangements; and that’s a positive; it’s how we move forward.
Despite this, there are obstacles on the road. Because these standards support a safety approach, they apply to both manufacturers and healthcare organisations (and also clinicians and patients, who have their own part to play in using and interpreting these tools safely).
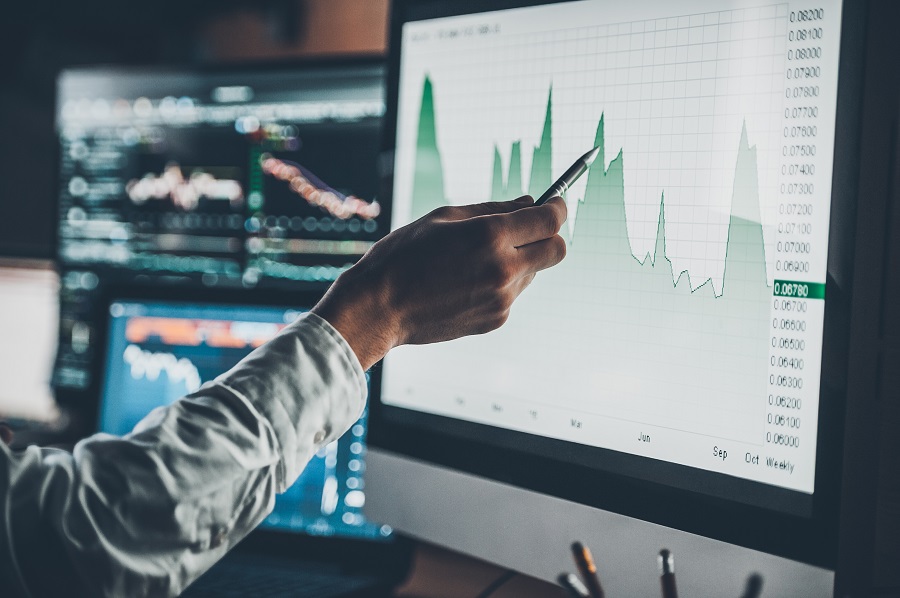
In theory, that means the cost of compliance should be borne by both manufacturers and users; but in practice there is considerable push-back from healthcare organisations against being asked to pay for something that is not mandatory.
Mandation may be coming. The UK government has a roadmap for the development of an effective AI assurance ecosystem, and the healthcare AI standards are part of it.
The EU has also adopted landmark legislation to create a legal framework for the development and adoption of AI, that covers data quality, transparency, human oversight, and accountability; and manufacturers who operate beyond the UK are not going to be able to ignore it.
Time for a proactive approach
We also need to make sure that healthcare organisations are proactive about using these standards and set-up to work with them.
That means making sure they have well trained, competent, clinical safety officers in place, but also making sure they are working within safety management systems that include everyone, from board to ward, in the design, development and deployment process.
I’m planning to write more about this later in the year. Meantime, the key point is that this is all about trust. If we want to build a healthcare AI industry in the UK, we need trust. If we want organisations (and the clinicians working in them, and the patients relying on them) to benefit from that industry, we need trust.
Raising awareness of the standards that are available for the development and adoption of digital health systems and AI tools is vital because they give us a structure and process on which to build that trust.
Everybody can see what has been done to make sure the development and deployment of these new technologies is ethical and clinically safe, and that will build confidence in the ability of AI to deliver a more accessible, efficient, and high-quality NHS.
Dean Mawson: Is a registered nurse working in London with an interest in clinical safety going back almost two decades. He is clinical director/founder at DPM Digital Health Consultancy, a company that providers regulatory compliance services to digital health manufacturers and health organisations.
Recent Comments